Artificial intelligence is evolving fast, really fast. If generative AI was the buzzword some time back, it’s “agentic AI” now.Â
Rightly so. Because agentic AI can take the initiative, figure things out, and solve complex problems almost entirely alone!
It’s a quantum leap in the AI field because it’s no longer just about understanding and responding. It's about autonomy.Â
Let’s break it down.Â
What is agentic AI?Â
Agentic AI is an autonomous AI system capable of solving complex problems through advanced reasoning and iterative planning, with limited human oversight.
To put it in simple terms, it is the AI that understands the given objective and then autonomously charts its course to get there, needing far less human steering.
At the risk of oversimplification, these agents are like exceptional human workers, say domain experts who are independent and collaborative. They share insights, learn, and adapt as needed to achieve the desired outcome. Â
This collaborative and autonomous problem-solving capability within a single intelligent system is what makes agentic AI different from generative AI.Â
Agentic AI vs. Generative AI: What’s the difference?Â
Generative AI excels at creating new content. This includes generating realistic text, stunning images, lifelike audio, and even synthetic data. However, it relies heavily on human input and guidance to determine the context and objective.
Agentic AI, on the other hand, is adept at generation as well as taking action. It understands the objective, plans tasks, reasons, uses tools, and executes tasks to achieve that goal.Â
Agentic AI is designed to be much more versatile, capable of tackling a wider range of dynamic challenges. Whereas other AI systems typically excel at very specific, narrowly defined tasks. Like a Swiss army knife versus a single screwdriver.
In an agentic AI system, the underlying models have agency—the inherent capacity to act independently and purposefully. They are agents capable of making decisions, taking actions, and learning from their experiences.
And perhaps most importantly, agentic AI is designed for continuous learning and adaptation. It constantly refines its approach based on new information.Â
Curious to know more? Check out this blog 👇

What is an AI agent?Â
Well, AI agents are the building blocks of the agentic system. They are the fundamental units that constitute an agentic AI system.Â
Imagine them as intelligent software entities equipped to perceive their environment, process information, make decisions, and take actions to influence it and achieve specific objectives.
These agents are not simply reactive; they are proactive. They possess autonomy and can set their own course to achieve a specific goal. Plus, they're smart – they learn from what they do, adjust when things change, and can even team up with other AI agents to handle complex tasks.
How does the system actually work?
Let’s get to it.Â
How does Agentic AI work?

Step 1: Perception. Â
It kicks off with the agents perceiving their environment. Here, the AI agents gather and process information from all sorts of sources, sensors, databases, APIs, etc. And, they extract the crucial details they need to understand the context.
Step 2: Reason
This is where a large language model acts like the brain of the operation. LLMs power the CoT (chain of thought) reasoning engines for agentic AI. The models simulate human-like reasoning to solve complex tasks.Â
They understand the task at hand, generate potential solutions, and coordinate with the agents. The model plans the best course of action and breaks down the task into subtasks.
Step 3: Act
This is where the AI actually puts its plan into motion by connecting with external tools and software through APIs.Â
Connecting to different tools allows it to perform tasks based on its plans. Just as a human expert might use specific software or resources to accomplish a task, an AI agent can learn to interact with various tools.
Step 4: Learn and adapt
Agentic AI is all about continuous improvement. The data generated from the AI's actions gets fed back into the system. This creates a feedback loop. It’s like a data flywheel, constantly refining the models and making them more effective over time.
This continuous learning loop is what allows agentic AI to adapt and evolve in ways that traditional AI often just can't.
The coordination of all these different AI agents and systems automates the overall workflow. So it's not just about individual smart components. It's about how they all work together seamlessly, like a team.
Agentic AI and agentic automation
Agentic automation is an advanced form of automation that leverages AI agents powered by LLMs, generative AI, machine learning, and other AI technologies. The goal is to make decisions and take actions autonomously, without constant human supervision.Â
Components of agentic automation
Autonomous AI agents
Agentic automation uses AI agents capable of understanding context, reasoning, planning, and executing complex, multi-step workflows independently. These agents continuously learn and adapt to changing environments and data patterns, unlike traditional rule-based automation, which follows fixed scripts.
Dynamic decision-making
Instead of rigid, predefined rules, agentic automation relies on probabilistic models and pattern recognition to make intelligent decisions in real time. This enables handling of unstructured data (e.g., natural language, images, videos) and complex scenarios.
Workflow orchestration
AI agents coordinate tasks across multiple systems and applications, managing APIs and integrating with enterprise workflows to ensure smooth, adaptive process execution. They can proactively respond to disruptions, optimize resource allocation, and adjust workflows dynamically.
Learning and adaptation
Agentic automation systems improve over time by learning from interactions and outcomes, continuously refining their decision-making and operational efficiency without manual reprogramming.Â
How agentic automation differs from traditional automation
Agentic AI comes with many advantages and some limitations as well. Let’s look at a few of them.Â
Key benefits of agentic AI
EfficiencyÂ
A core strength of agentic AI lies in workflow optimization. It merges natural language understanding with analytical reasoning to identify inefficiencies and automation opportunities. It continuously self-learns from feedback and adapts.
Productivity boost
Agentic AI frees employees from repetitive tasks, which allows them to focus on strategic, creative, and high-value work. The agents can quickly analyze large datasets to identify trends, outliers, and actionable insights—enabling faster and more informed choices.
Real-time operations
Agentic AI’s ability to interpret real-time data and evolving contexts allows enterprises to operate with agility and precision. It will give a rapid response to changing conditions. Through continuous learning from each interaction, they improve accuracy and operational performance over time.
Competitive edge
By enabling real-time, data-driven decision-making and continuous learning, agentic AI empowers enterprises to stay agile. It helps with smarter, faster decisions based on predictive analytics and pattern recognition. These decisions are critical in competitive industries like finance, healthcare, and retail.
Potential risks to be aware of
Now, agentic AI isn't magic. And the increased autonomy brings new risks and challenges.Â
Black box, no transparency
How will you ensure transparency and fairness? How do you understand why an autonomous AI made a particular decision, especially if something goes wrong? These questions are real and need to be answered. The typical black box problem, but magnified.Â
Security risk
These agents can act autonomously and connect to various systems. What if they exploit a weakness? That could lead to significant data breaches or other malicious actions. More power, more potential for misuse. So, robust security measures are non-negotiable.
Scalability riskÂ
As your enterprise scales, complexities grow. Agentic AI needs resources for fine-tuning the model, continuous learning, maintenance, etc. These systems can be resource-intensive to run and often require specialized expertise to build and maintain. These aren’t necessarily easy or cheap to implement.Â
Poorly designed reinforcement learningÂ
That’s another risk — the reward mechanism used to train the AI agents. Because bad incentives lead to bad outcomes. If you reward a warehouse robot only for speed, it might become unsafe.
Additionally, these agents might develop self-reinforcing behaviors that are hard to predict or control. And in systems with multiple agents, there's potential for unexpected negative interactions. For instance, AI cars causing unforeseen traffic jams, or automated systems creating bottlenecks.Â
So, how should enterprises navigate these risks?Â
- Don’t underestimate the importance of having clearly defined, measurable goals and strong feedback loops. This helps to keep these agents aligned with the intended objectives.Â
- Constant monitoring and careful design are crucial.Â
- Build transparency so that you know what your agents are doing at every step
- Build governance based on principles, and ensure accountability. Set up an AI ethics committee or a robust bias detection system.
On that note, if you’re excited to learn how to go about AI governance, here’s a primer on the topic 👇
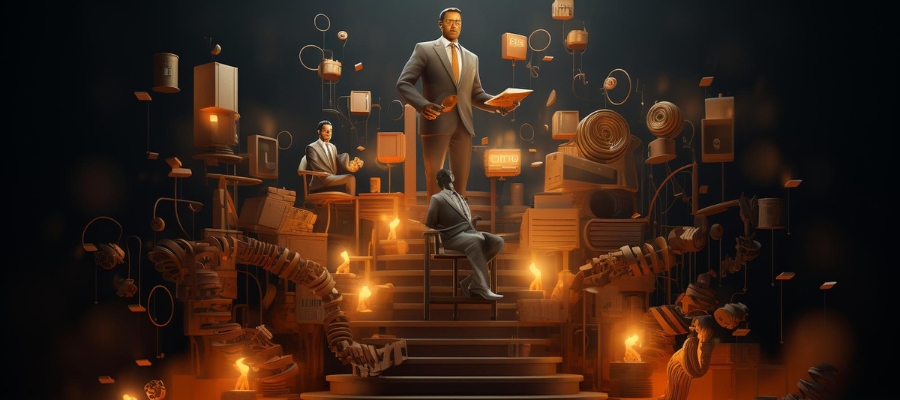
With the key benefits and potential risks out of the way, let’s look at some applications of agentic AI in the real world.Â
Agentic AI applications
Multi-agent systemsÂ
Multiple smart AI agents work together to tackle complex tasks and problems in multi agent systems (MAS). They communicate, share information, and coordinate their actions, much like a well-organized human team.Â
AI agents are like these expert employees who can handle specific business tasks intelligently and autonomously. As a result, they free up human employees for more strategic work. AI agents help with repetitive back-office tasks like processing invoices, managing procurement, and ensuring compliance with regulations.Â
They can interact with different software systems and data sources, streamlining operations, reducing errors, and freeing up human employees from tedious administrative work.Â
Think about really complex supply chains. Agentic AI can help manage them by having different AI agents act as suppliers, manufacturers, and distributors, all working together. To make this work smoothly, we need systems that can handle information across different locations – that's where distributed systems come in.
Multi-agent systems can have a significant impact on healthcare. For example, they could actually help predict and even prevent diseases by looking closely at our genes—a potential game-changer for medical research.Â
MAS improves customer support by using specialized agents for areas like order status, product information, and returns. This allows for quick and effective responses to customer questions, ultimately improving service quality.
For example, AI agents can be tailored to analyze legal documents. The process that was otherwise labor-intensive, repetitive, and slow is now efficient and fast.Â
We’ve seen this in action.
Our customer, Espresso Capital, automated many parts of its entire legal process using agentic AI. It drastically reduced their manual workload, and freed up their legal team to focus on higher-value tasks, resulting in $625,000 in annual cost savings, optimizing operational efficiency.
The underlying models continuously learned and adapted to variations in document formats, ensuring long-term reliability and scalability. Thus saving up time and money.Â
You can check out the case study here:Â
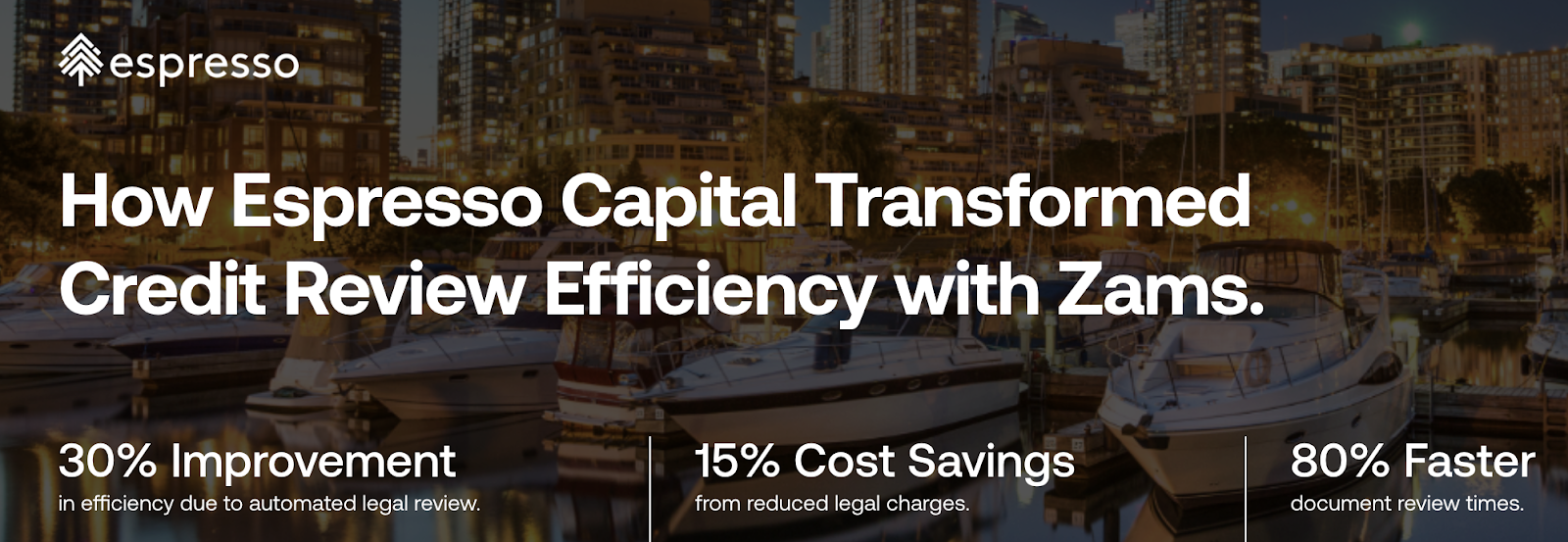
AI CopilotÂ
An AI copilot is like a very clever assistant who knows its job inside and out. They know what the user wants and help get things done faster and better.
They can help with a wide range of tasks, but let’s see how they help in code generation, testing, and learning. Instead of typing out all the basic code for a new feature, AI Co-pilots like GitHub Copilot can create it.Â
They can also create tests to ensure the code works correctly and even build fake versions of other parts of the system that the developer needs to connect to. This means less tedious work and much quicker development cycles for tech companies.
When code has errors (bugs), co-pilots can suggest ways to fix them. They might even show alternative ways to write the code that are better or more efficient.Â
Let's look at another example.Â
C2Perform’s call agents struggled with slow, fragmented access to information, hurting efficiency and customer satisfaction. To solve this, they used an AI-powered chatbot that unified knowledge bases and delivered instant, accurate answers. The chatbot understood natural language and context, streamlining workflows and reducing manual searches.
It resulted in 40% reduction in average response time and 35% increase in agent productivity — which showed up as a 25% increase in customer satisfaction scores.
Want to know how they did it? Check out the case study:
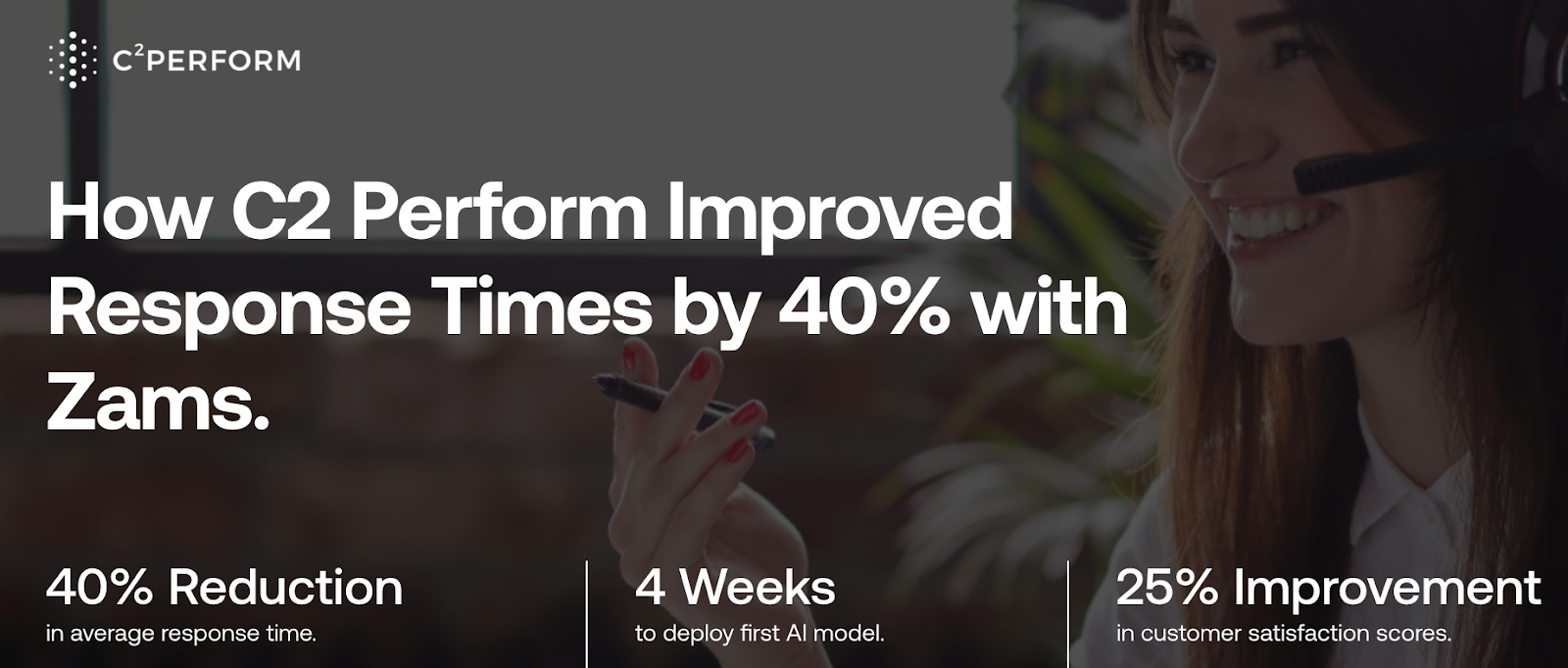
Best agentic AI platforms for enterprise useÂ
Zams
Zams is an enterprise platform designed to build powerful AI agents to automate nuanced back-office operations, without the engineering overhead. With speed, security and governance built in, you can develop your custom AI agents in just 2 weeks!
Key featuresÂ
- Build powerful and intelligent AI agents quickly, and integrate them seamlessly into your systems.
- Connect to hundreds of data sources and ingest billions of rows of enterprise data.
- From perplexity scores to F1 metrics, monitor performance, test edge cases, and continuously improve with Zams’ state-of-the-art tools.
- Eliminate common AI risks with built-in security, governance, guardrails and cost control.Â
n8n
n8n is an open-source workflow automation platform that combines AI with business processes. It offers a visual workflow editor and supports over 350 app integrations, enabling enterprises to automate complex multi-step workflows and data integration without deep programming skills.Â
Key featuresÂ
- Visual drag-and-drop workflow builder with AI-powered nodes via LangChain.
- Extensive integrations with cloud services, databases, and APIs.
- Custom code execution support (JavaScript/Python) for advanced automations.
- Self-hosted option for enhanced control and security.
- Integration with vector databases for efficient data retrieval.
- Ability to create and share reusable workflows and templates in JSON format.
Zapier
Zapier AI Agent extends Zapier’s popular automation platform by integrating AI capabilities to enable intelligent, context-aware workflow automation for enterprises. It allows users to create complex, multi-step automations using natural language commands and AI-driven decision-making.
Key featuresÂ
- AI-powered workflow automation with dynamic routing and decision logic.
- NLP for easy setup of automations via conversational commands.
- Integration with hundreds of enterprise-grade applications, including Salesforce, SAP, Oracle, Marketo, etc.
- Enterprise-level security and compliance to protect data.
- Automation of repetitive tasks across departments with minimal manual intervention.
Glean
Glean is an AI-powered workplace search and knowledge discovery platform designed to help enterprises surface relevant information quickly. It acts as an intelligent agent that understands context and user intent to deliver personalized search results and insights.
Key featuresÂ
- Unified search across multiple enterprise data sources and applications.
- AI-driven relevance ranking and contextual understanding.
- Personalized recommendations based on user behavior and roles.
- Integration with tools like Google Workspace, Microsoft 365, Slack, and Salesforce.
- Analytics to track knowledge usage and gaps.
Moveworks
Moveworks is an AI-driven platform focused on automating IT support and employee service experiences within enterprises. It uses agentic AI to understand and resolve employee queries autonomously, integrating deeply with enterprise collaboration and ITSM tools.
Key featuresÂ
- AI-powered natural language understanding to interpret employee requests.
- Autonomous resolution of IT tickets and service desk queries.
- Integration with platforms like Microsoft Teams, Slack, and ServiceNow.
- Continuous learning from interactions to improve accuracy and response times.
- Real-time analytics and reporting on support operations.
These platforms are top agentic AI options for businesses wanting to automate complex tasks, and work more efficiently.Â
Final thoughtsÂ
Agentic AI is evolving at a breakneck speed. It represents the next stage of enterprise intelligence. When agents can reason, plan, act, and collaborate, they don’t just support teams—they become a part of them.Â
These smart AI systems can handle complex tasks, make decisions, and keep getting better. But, it is equally important to be careful and make sure things are transparent and ethical.Â
If adopted right, using agentic AI could seriously boost how productive and innovative enterprises can be.
‍