We’re witnessing a fascinating shift in artificial intelligence. One that moves beyond content generation toward autonomous action.
Generative AI (gen AI), like GPT or Claude, has shown the world what machines can do with language, image, and code generation. But that has now led us to ask “Great! But what’s next?” And more importantly, “How do we turn all this AI output into a real, operational outcome?”
That’s where agentic AI comes in.
If gen AI retrieves and generates a response to us contextually, agentic AI takes it to the next level by getting thighs done. It takes the information and leverages it into a process to drive an outcome. Agentic AI is capable of reasoning, taking initiative, and executing tasks across systems without constant human prompts.
In this article, we will break down in detail, the difference, their goals, limitations, and use cases.
Let’s dive in.
Is Agentic AI just a more autonomous form of Generative AI?
Not quite.
Generative AI is a type of artificial intelligence that creates novel content based on patterns learned from existing data. It uses deep learning models to create content—text, images, video, audio, and even software code—with a prompt. It learns from huge piles of data and generates content by identifying patterns within that data.
While agentic AI builds on generative models, it's more than just a smarter chatbot or a better text generator.
Agentic AI operates autonomously by interpreting inputs, making choices, and executing actions independently of human guidance. This agent-based approach allows AI to move beyond singular tasks and help with a wider variety of tasks.
For example, AI agents can be used in complex tasks like invoice processing, handling customer requests, and making product recommendations.
Where generative AI excels at generating content, agentic AI is designed to act. It makes decisions, takes initiative, works toward goals, and interacts with tools, data, and humans.
Now that you know what gen AI and agentic AI are, let’s look at some important differences between them.
TL;DR: Agentic AI vs Generative AI
The differences between them are:
What’s the key difference?
Agentic AI doesn’t just generate, it acts. Generative AI creates content based on prompts, while agentic AI takes action to meet the goal by reasoning, using tools, adapting, and executing tasks autonomously.
Agentic AI can perceive data from various sources, reason through it, act on it, and learn from the outcomes.
Here’s the four-step process (perceive, reason, act, learn) enables it to tackle challenges that gen AI cannot address due to its reactive nature 👇
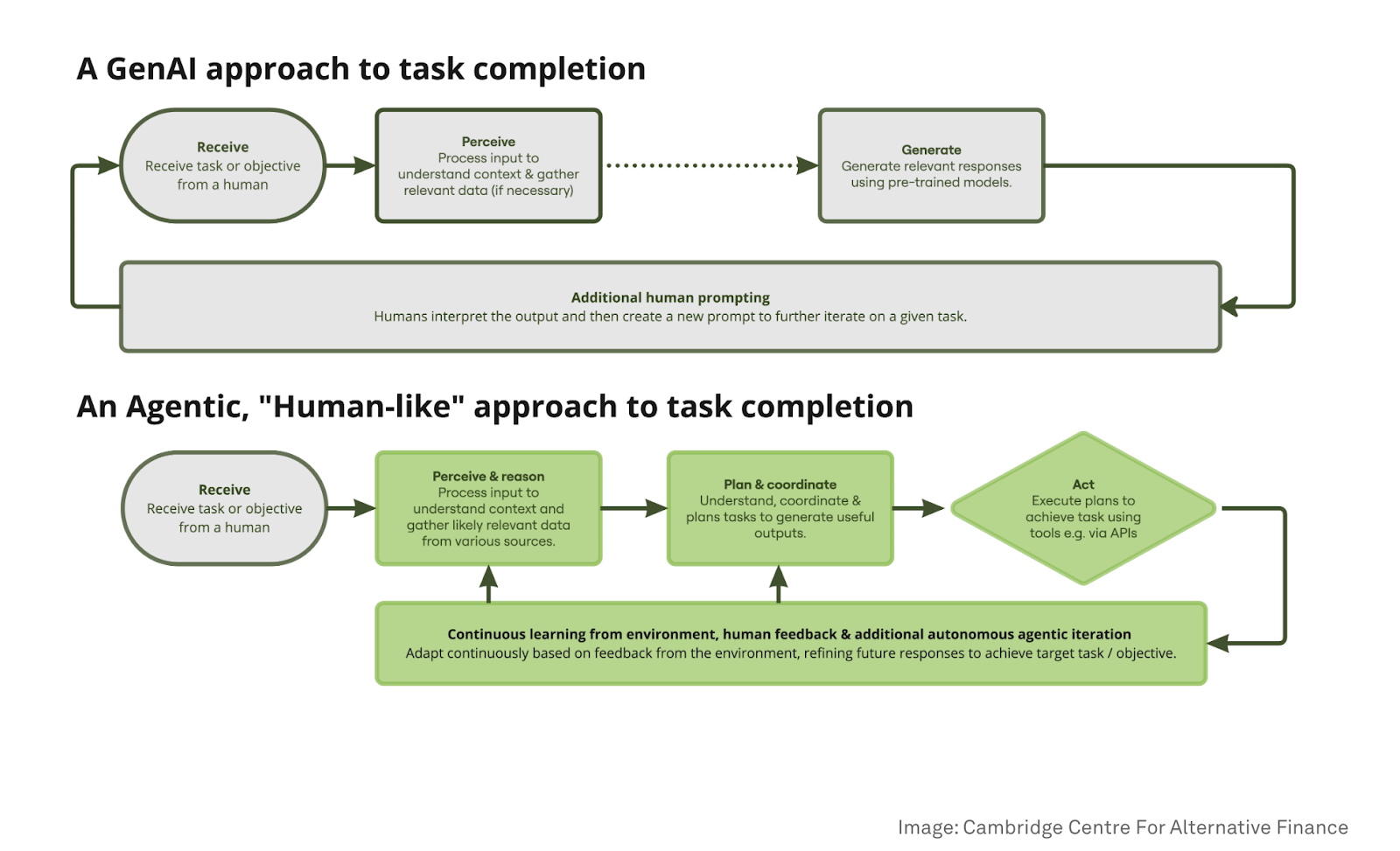
Goals of Agentic AI vs the goals of Generative AI
The goals of agentic and generative AI differ fundamentally, reflecting their distinct functionalities and applications within broader AI.
Generative AI goals
1. Enhancing content productivity
One of the first things you can achieve using Gen AI is producing high volumes of content quickly, i.e., you can go from brainstorming to drafts to edits and publishing — including content tailored to specific audiences and use cases. It helps save time and effort for creators, marketers, researchers, and professionals, and more.
2. Enhancing user experience
Generative AI also helps you enhance user experiences by providing tailored content and solutions. By analyzing user inputs and preferences, it can generate personalized recommendations, automate creative processes, and improve engagement across various platforms.
3. Support decision making
While gen AI isn’t primarily focused on decision-making, it can assist in this area by providing insights and generating data that inform business strategies.
For example, it can create reports or visualizations that help organizations understand trends and make informed decisions.
Agentic AI goals
1 Autonomy and decision making
Agentic AI helps you run operations autonomously, where it makes decisions and takes actions without the need for constant human oversight. Imagine running complex workflows that involve working towards specific objectives, and adapting to constant environmental and contextual changes.
2. Refining strategy and performance on the go
Agentic AI solves complex problems by continuously learning from interactions and adapting its strategies based on real-time data. Its ability to learn from interactions and experiences enables it to continuously update its knowledge, refine its strategies, and enhance its overall performance.
This capability allows it to handle dynamic environments effectively, making it suitable for applications in areas like robotics, customer service, and supply chain management.
3. Workflow automation
Another key goal is to automate and optimize workflows. Automation helps reduce manual effort and improves productivity. By integrating with existing systems, agentic AI can streamline processes, manage tasks, and optimize operations, ultimately leading to cost savings and increased efficiency for organizations.
Limitations of Generative AI that Agentic AI addresses
Generative AI is great at producing content, but it begins to fall short where things get complex. It doesn’t take initiative, remember the context, or handle multi-step tasks without constant prompting.
Also, the output is only as good as the data it learned from. If the data has biases, isn’t clean, or is incomplete, the AI's output will mirror those exact issues.
GenAI is incredibly good at pattern matching and prediction. It predicts the next word or pixel, or whatever, based on what it's seen before. But, probably not reliable when it comes to real comprehension.
And the biggest pet peeve, among all users we bet, is about hallucinations.
GenAI states things that just aren't true, so confidently. It often misleads users into believing those things. Plus, there is this transparency issue. GenAI is not always clear how it got to an answer.
So, how does agentic AI address these limitations?
Agentic AI goes beyond just answering, it executes. Agentic systems bring that loop of perception, reasoning, and action. It can trigger tools, make decisions, and adjust based on real-time feedback.
If you’re deploying AI in real operations, you need more than answers—you need execution. Agentic AI enables collaboration between multiple agents, facilitating the development of intricate workflows.
On that note, if you want to go deeper into how these AI agents work, with real-world examples, you might to check out our primer on the topic 👇
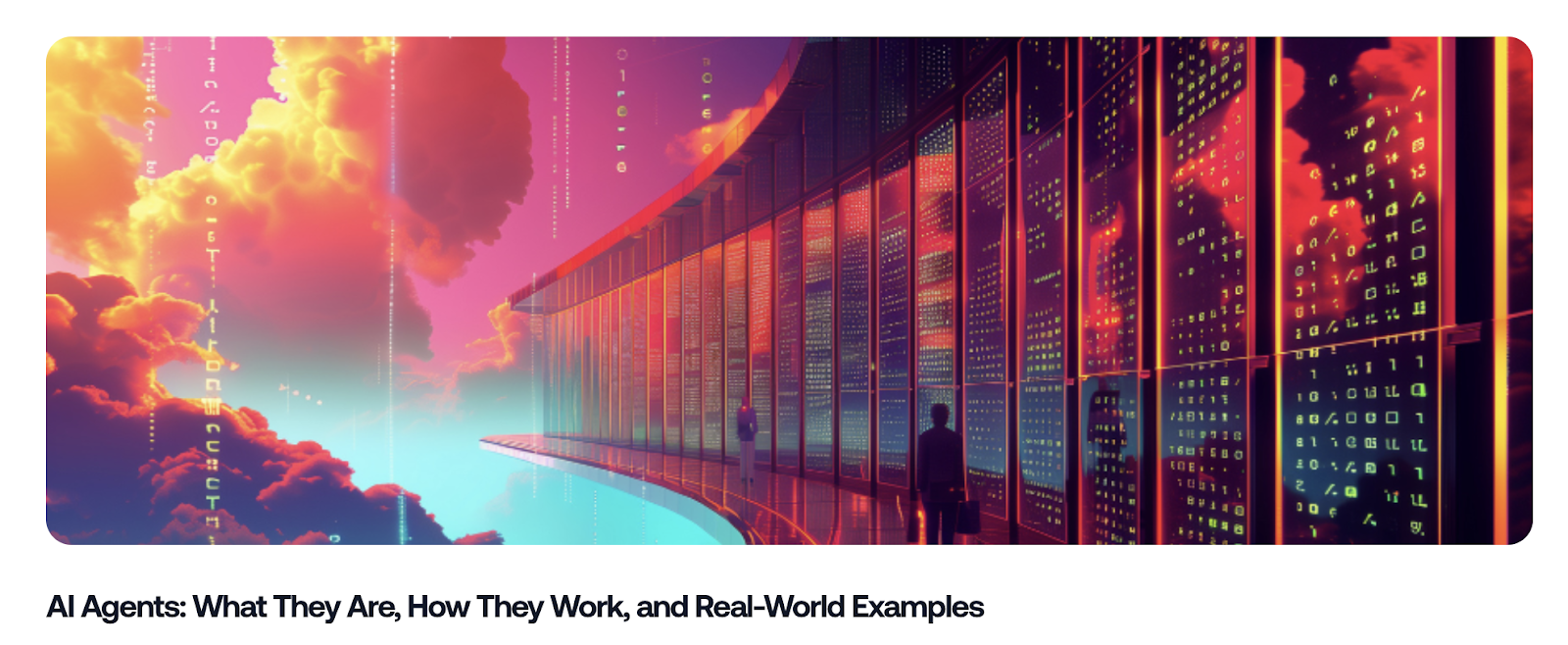
How does Agentic AI expand on the capabilities of Generative AI?
Generative AI is a robust tool that helps various professionals, including creatives, engineers, researchers, and scientists. For instance, generative AI can transform text into an image, an image into a musical piece, or video content into text.
But businesses need more than just an AI that responds; they need systems capable of independently handling and completing intricate workflows and tasks. They need enterprise AI that can proactively predict, strategize, and act autonomously to meet their objectives.
This is exactly what agentic AI does.
It significantly expands the capabilities of generative AI by introducing autonomy, complex problem-solving abilities, and enhanced workflow automation.
Its ability to learn and adapt in real-time allows it to operate effectively in dynamic environments, making it a powerful tool for organizations looking to innovate and improve operational efficiency.
Let’s look at their features in detail.
Gen AI features
Content creation
Generative AI excels at producing new and original content, including text, images, audio, and video. It can generate coherent and contextually relevant outputs based on user prompts, mimicking human creativity.
Training data
This type of AI relies on large datasets to learn patterns and structures. It uses advanced algorithms, such as generative adversarial networks (GANs), to create outputs that reflect the features of the training data.
Human-like interaction
Generative AI can engage in natural language processing, allowing it to produce human-like text and responses. This capability makes it valuable for applications like chatbots and virtual assistants, enhancing user interaction.
Adaptability
Generative AI can adapt its outputs based on the context and nuances of the input it receives. This flexibility allows it to generate tailored content that meets specific user needs or preferences
Agentic AI features
The key features of Agentic AI are:
Autonomy and decision making
Agentic AI functions autonomously, driven by its own objectives. It determines and executes necessary actions to achieve these goals without requiring human intervention.
Problem solving
Agentic AI solves problems through a four-stage process: perception, reasoning, action, and learning. First, AI agents collect and process data. Next, an LLM orchestrates the analysis of this perceived data to comprehend the situation. Finally, the AI collaborates with external tools, continuously improving and learning from feedback.
Learning
Leveraging advanced natural language processing techniques, agentic AI comprehends human language at a deep semantic level, facilitating rich interactions.
Workflow optimization
It combines language understanding with reasoning and decision-making to optimize business processes, enhance resource allocation, and identify automation opportunities.
Multi-agent systems
Agentic AI can collaborate with multiple agents, tools, etc, which enables it to create multi-agent systems and complex workflows. It can connect with different systems and tools to execute various tasks.
For instance, in research, it can collect data, analyze findings, and create reports, potentially working with other AI agents to improve results. This interconnectedness allows AI systems to collaborate and achieve shared objectives.
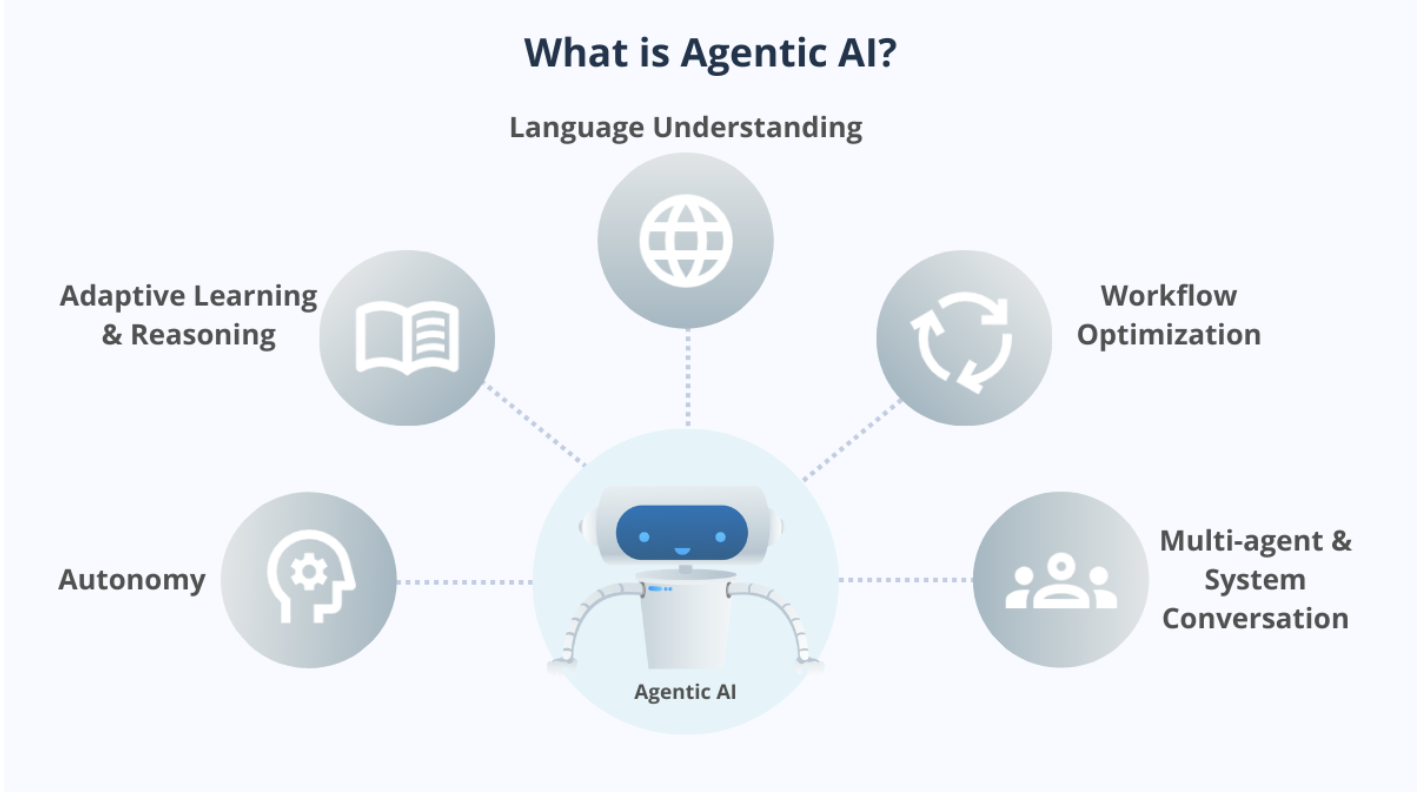
While Generative AI excels in content creation, agentic AI's capabilities extend to various domains, including cybersecurity, healthcare, and complex data analysis.
For example, it can autonomously detect and respond to security threats or manage patient interactions in healthcare settings, showcasing its versatility beyond mere content generation.
Agentic AI vs generative AI use cases
Both have diverse use cases where their applications are very helpful. Let’s look at a few of them.
Gen AI use cases
1. Content creation
Gen AI excels in producing creative content, such as marketing materials, social media posts, and even scripts for videos. It can generate high-quality visuals and text based on user prompts, significantly reducing the time and resources needed for content production.
2. Customer interaction
In customer service, it can power chatbots that provide immediate, context-aware responses to customer inquiries, enhancing user experience and reducing the workload on human agents.
3. Software development
Generative AI assists in coding by generating code snippets from natural language descriptions, helping developers accelerate the coding process and improve productivity.
4. Marketing
In marketing, gen AI can create personalized email campaigns and promotional content tailored to specific customer segments, enhancing engagement and conversion rates.
5. Research and development
Generative AI helps in R&D by generating hypotheses, analyzing data trends, and even creating synthetic data for testing new products or services, thereby speeding up innovation cycles.
Agentic AI use cases
1. Cybersecurity
Agentic AI enhances security by autonomously monitoring systems for vulnerabilities and responding to threats in real-time, outperforming traditional security measures
2 Portfolio management
In finance, it can manage investment portfolios by analyzing market data, making buy/sell decisions, and adjusting strategies based on market conditions, thus automating complex financial processes.
3 Healthcare
In healthcare, agentic AI can monitor patients, manage appointments, and assist in diagnostics by analyzing patient data to suggest treatment options. Hence, improving patient care and operational efficiency.
4 Customer experience
Agentic AI, through its agents, can autonomously handle complex customer inquiries. It can learn from interactions to provide personalized solutions. For instance, it can analyze live shipping data, determine the cause of delays, and offer resolutions like expedited orders or refunds without human intervention.
5. Supply Chain Management
Agentic AI optimizes supply chains by predicting risks, managing inventory, and dynamically adjusting logistics based on real-time data, which helps in maintaining resilience against disruptions.
6. Loan Processing
AI agents expedite loan approvals by automatically reviewing applications, verifying applicant details, and requesting missing documents.
AI agents can automatically review loan applications, verify information, check creditworthiness, and approve them if they meet the criteria, speeding up the process and improving customer satisfaction.
7. Virtual R&D Assistants
AI-powered virtual R&D assistants greatly reduce the time researchers spend finding relevant papers, patents, and technical documents from large databases, speeding up innovation. These agents can assess findings, identify gaps, and even propose new ideas based on data patterns.
Some advanced systems can simulate research or product testing, allowing teams to analyze results before real-world trials. This reduces the cost and effort of R&D, improves decision-making, and accelerates discovery.
It’s a wrap
So let's pull this together.
Generative AI is the content creator. It generates text, images, writes code, and responds to prompts. On the other hand, agentic AI is the autonomous actor. It makes decisions, takes actions, and proactively works towards goals.
Both AI types have different learning styles and interaction modes. But they can work together powerfully, especially in areas like customer service. Looking forward, agentic AI is pegged as strategically important in the coming years, promising big productivity boosts.
But both come with significant challenges. Bias, safety, security, and potential for misuse need careful management and ethical consideration. It feels like we're moving towards AI systems that are much more autonomous and proactive.
Agentic AI seems poised to really change how we interact with technology and how our businesses run.