Ever wonder what it'd be like to run a business where things just...flow? Like your supply chain figures itself out, bugs get fixed before anyone even notices, and your whole operation just hums along perfectly in the background?
It is happening now. By weaving AI agents into their core processes, businesses already leverage AI for smooth workflows and faster, autonomous decision-making.
If you’re still ‘experimenting’ with AI, you run the huge risk of falling behind. But worry not, this post will help you cut through all the noise and get a solid handle on what enterprise AI actually means.
We'll unpack why it's essential now, clear up some common misunderstandings, look at the challenges, and how to adopt it.
So, let’s dive in.
What is enterprise AI, really?
Enterprise AI is about embedding intelligence deeply and strategically into how an enterprise runs—its core operations.
It’s like systems that don't just record information, but actually analyze it, make smart choices based on that analysis, and then act on them. So it's not just about plugging in one AI tool here or there; it's broader.
It's a holistic approach, where you embed AI capabilities across the layers of your entire organization, at scale.
Until now, we have often seen AI to be used for specific, isolated tasks, almost in silos. Whereas the idea of enterprise AI is to be able to handle complex company-wide stuff, in an integrated, cohesive environment with complete context.
Getting started with enterprise AI solutions
Enterprises are no longer questioning whether to adopt AI, but rather how to strategically integrate it into their existing frameworks to unlock tangible benefits.
A well-defined and structured approach is crucial to navigate the complexities of adopting AI, ensuring that initiatives are aligned with overarching objectives of the enterprise, maximize potential gains, and effectively mitigate inherent risks.
Several leading organizations across industry domains are already leapfrogging by leveraging multi-agent frameworks to build their AI agents to solve high-impact problems, streamline and scale operations, and more.
Few examples include:
- GM's OnStar - They're using Google Cloud's conversational AI for a more natural virtual assistant experience.
- Mercedes-Benz is using Google Cloud's automotive AI agent for better voice search and navigation in its cars.
- And then you've got UPS building a digital twin of its entire distribution network, using AI to optimize logistics.
And mind you, AI can't just be an IT thing, it's got to come top-down. Why?
If AI stays stuck in IT or data science, it might just stay experimental forever.
So leadership buy-in and driving it are non-negotiable. Without it, you just won't unlock the real potential.
Driving adoption in the enterprise AI world
Let’s look at 7 key steps that can act as your framework to successfully drive enterprise AI adoption, transforming your operations like never before.
1. Evaluating the right models for your use case
When you're thinking about bringing AI into your enterprise, the very first thing to do is figure out ‘why.’ It's not just about having the latest tech; it's about hitting real business goals.
Not the vague questions. Ask for specifics.
For example: Can I accurately predict additional accessory fees that not only leads to revenue leakage, but is also making impacting my operational forecasting difficult and impacting profitability?
On that note, you might want to check this case study:

So, it often starts with taking a good, hard look at where things are inefficient, where you could grow, and how AI could — not only give you insights but also execute, making an operational impact.
You need people from all over the company talking about what they need and what's important.
When you're checking out enterprise AI models, it's not enough to just look at the flashy numbers. You've got to see how they perform on the stuff that actually matters to you and your customers, and you definitely need to make sure they're secure and play nice with your current systems.
So, choosing the right model evaluation framework becomes extremely important. Failing to follow this crucial step will lead to complications in the next stages.
2. Embedding AI in your daily operations
Everything starts with clarity on the purpose and outcome — and then investing on the infrastructure in ways that’ll help you grow.
Once you identify the opportunity, you know where exactly to embed AI in your products and processes to unlock capabilities and transform user experiences.
One of our customers, Husk, noticed that inaccurate energy consumption predictions resulted in inefficiencies in grid operations and pricing strategies.
So they wanted to accurately forecast energy consumption across its grids in India and South Africa, because they knew that a clear forecast could help them scale operations effectively, optimize grid pricing, and negotiate favorable energy contracts – much better and have greater control.
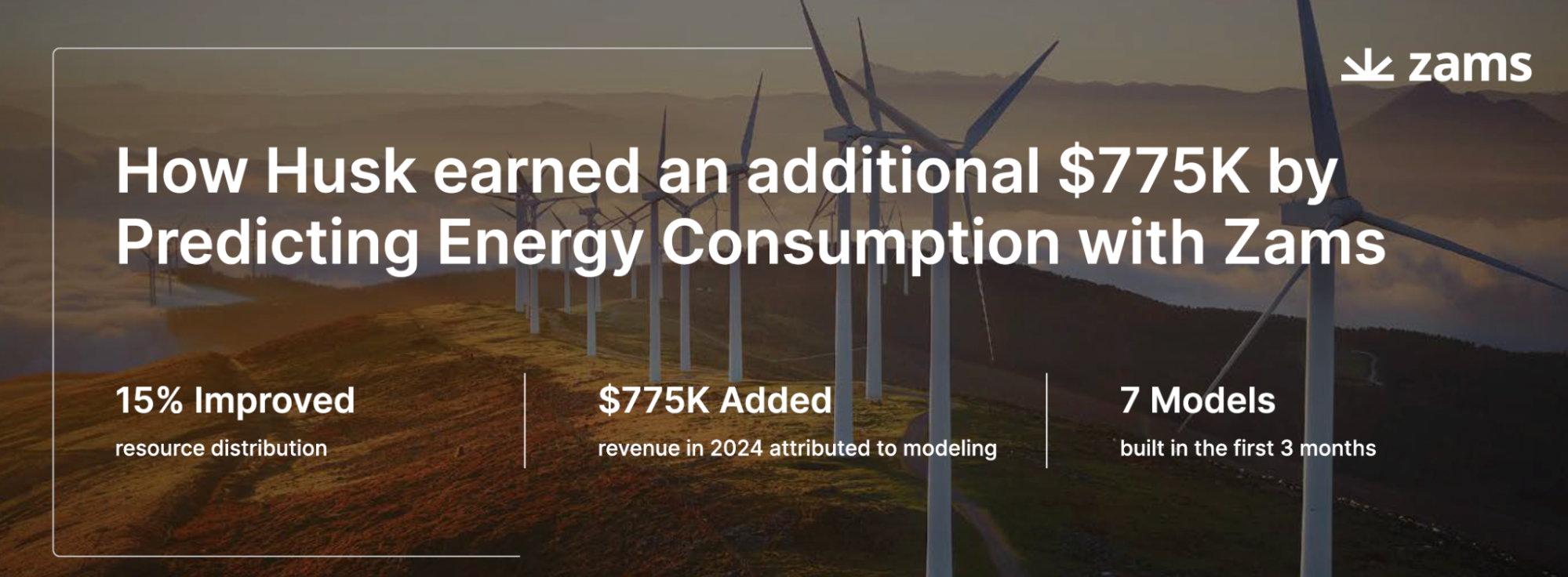
Embedding AI in the right areas, be it knowledge, process, or prediction – you’ll notice improvement across functions, including in customer interactions.
You can see your quick wins in the form of faster response times, handling routine inquiries, tailored recommendations, anticipating needs, and more — ultimately fostering a deeper sense of customer loyalty and engagement.
3. Start now to enjoy the compounding effect
Getting in early is the key because implementing an AI agent is not a start-stop plug-and-play solution. Models perform better over time as they iterate.
As you can see in the previous example of Husk, we built 7 models in 3 months
But to specifically talk about starting early and seeing a compounding effect, let’s look at the case of Creditt, which operates on a massive scale.
Creditt receives a massive flow of 20,000 loan applications per month. Each application takes 20 minutes to process manually, leading to a monthly backlog of 18,000 loans.
We helped them automate the loan application evaluation process using Zams, and over time, we saw that the time required to process each application went down from 20 minutes to just 5 minutes.
Talking of compounding, the approval workflow streamlining eliminated over 208 calendar days of backlog per month, significantly improving operational efficiency.

Long story short, organizations that start using AI now, even in small ways, learn faster and get better at it quicker — creating a snowball effect, making it harder for those who wait to catch up later.
Research from McKinsey highlights a significant long-term opportunity, estimating a potential $4.4 trillion in added productivity growth stemming from the corporate application of AI technologies.
Like they say, the best things in life are a result of compound interest.
4. Fine-tune your models for use cases
You can't just take any pre-trained AI model off the shelf and expect it to work perfectly for your enterprise.
It's like buying a suit — you usually need to tailor it to get the best fit.
Same with enterprise AI. You need to understand what your people need and then train the AI using data that's relevant to those needs.
Why? You need to ensure domain expertise, consistency, relevance, accuracy, and more.
Don't just look at the fancy overall scores of AI models; dig into where they make mistakes and where they fall short for your specific problems. That way, you can focus on training it in the areas where it needs the most help.
Playing around with different prompts and tweaking the AI also makes a big difference. And if you've got data sitting in other places, you can even teach the AI to pull that in to make it even smarter.
Ultimately, spending the time to customize AI for your enterprise can give you a real leg up and make sure you're actually getting your money's worth.
You can also think about building your own models. It makes sense if you think about it. Your data, your team, your use cases—everything is yours. But, for building your model, a team of data scientists should be at your disposal.
If you don't want to have engineering overheads, and yet want to get your AI agent up and running quickly, you need an enterprise AI orchestration platform like Zams.
5. Leverage your in-house experts
Getting enterprise AI right isn’t just about the tech team doing their thing. It’s about bringing together the people in your organization who are closest to the problems and processes you’re trying to solve.
Everyone needs to be on the same page about using AI responsibly and ethically. That means training on the rules and making sure everyone feels like they own a piece of it.
For instance, Espresso Capital got their team together in building an AI-powered question-answer interface that helped them save 1,250 lawyer hours annually, and also significantly reduced manual workload. By automating document analysis, they achieved $625,000 in annual cost savings, optimizing operational efficiency.

It's like their business smarts combined with AI's brainpower leading to true efficiency — which is way more valuable than if it were just built in a tech vacuum.
6. Unblock your developers
So, when we're talking about adopting enterprise AI, a big piece of the puzzle is how quickly your developers can actually get it up and running.
Do your developers have the bandwidth? Or are they working on some other priority?
But think about this for a second:
When you have a burning operational problem to fix — causing inefficiencies such as revenue leak or customer churn, and you know that fixing it can save/make millions of dollars — should the engineering bandwidth be an impediment to implementing enterprise AI?
It is in these situations, you can leverage an AI orchestration platform like Zams to build the agents you want, without having to spend a lot of engineering time.
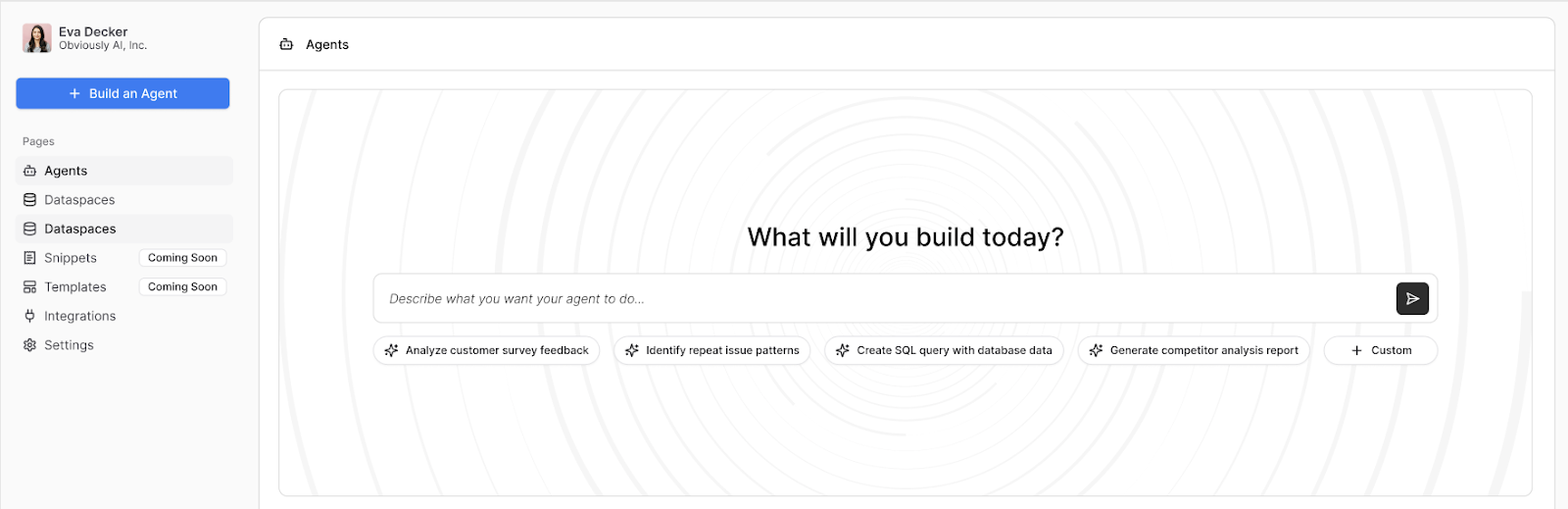
The main idea here is to take away some of the roadblocks so that you can get to adoption and outcomes faster.
7. Have clear goals for processes, automation, and workflows
Going big on automation isn't a walk in the park. Improving efficiency starts with absolute clarity on what to automate, what the nuances of those operations are, what an ideal workflow looks like, and then finding ways to automate it.
Plus, there are a lot more parameters to think about, like data privacy, ensuring your AI is unbiased, etc.
However, if done well, AI automation can touch almost every part of your enterprise, making things more efficient, productive, and saving money.
Wrapping up…
All set to make your enterprise an AI-powered organization? Great! But remember, the best AI deployment happens in an organization that retains the open mindset, supported by iterations and experimentations, rigorous evaluations and safety guardrails.
Start with why you need AI, then embed it into your products to truly deliver value for your customers. It's also about jumping in sooner rather than later, and making sure the AI models are a perfect fit for what you do.
Don't forget to lean on your in-house experts – they know the business best! Making it easier for your developers to work with AI is crucial too, and finally, aiming for some big automation wins can be a game-changer.
Think of these steps as connected pieces. You need to think about the goals of your enterprise and any potential risks. Picking the right way to measure if your AI is working is super important, and getting your team on the same page is key.
If you take a step-by-step, thoughtful approach, you can really make enterprise AI work for you, boosting innovation, making customers smile, and staying ahead of the curve.